The Siegel Lab has developed an undergraduate-friendly enzyme design-build-test workflow, which has been the scaffold for a successful training in the lab and has been further developed into a freshman Course-based Undergraduate Research Experience (CURE) at UC Davis. The central research goal of this workflow and project probes the current predictive limitations of protein modeling software by functionally characterizing single amino acid mutants in a robust model system.
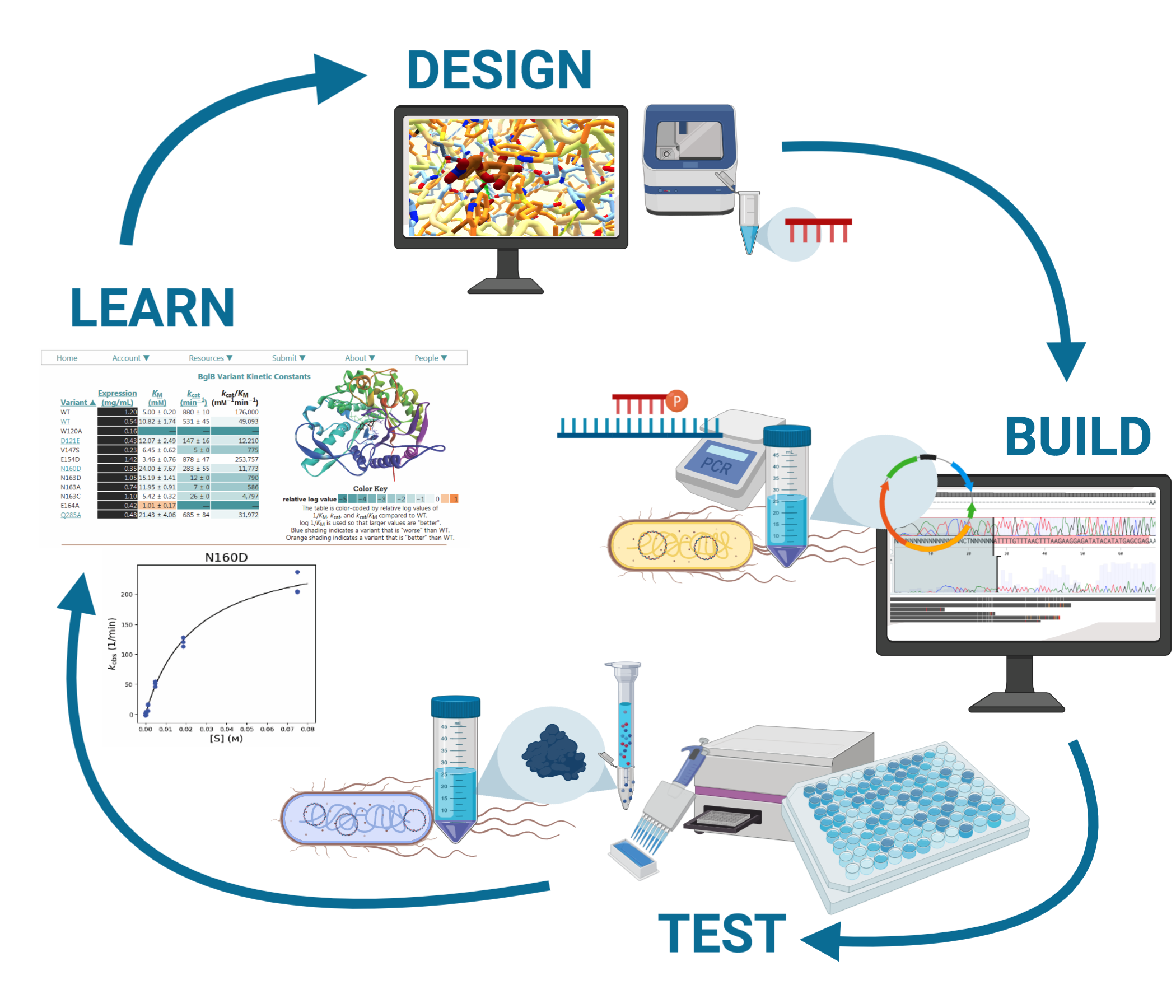
Undergraduate Research in the Siegel Lab
Through the Design2Data Program, undergrads learn basic molecular biology and biochemistry techniques and skills while they develop their knowledge of the lab's scope in enzyme research. Undergraduates have opportunities to present their work and are encouraged and guided through the process of writing a bioarchive paper, summarizing their results. Undergrads, if you are interested in applying to the lab, please checkout out our Undergrad Research resource page.
D2D Course-Based Undergraduate Research
The Data2Design project has been adapted as the First-Year Seminar Course-Based Undergraduate Research Experiences (CUREs): Making a Mutant. More information about CUREs at UC Davis can be found here!
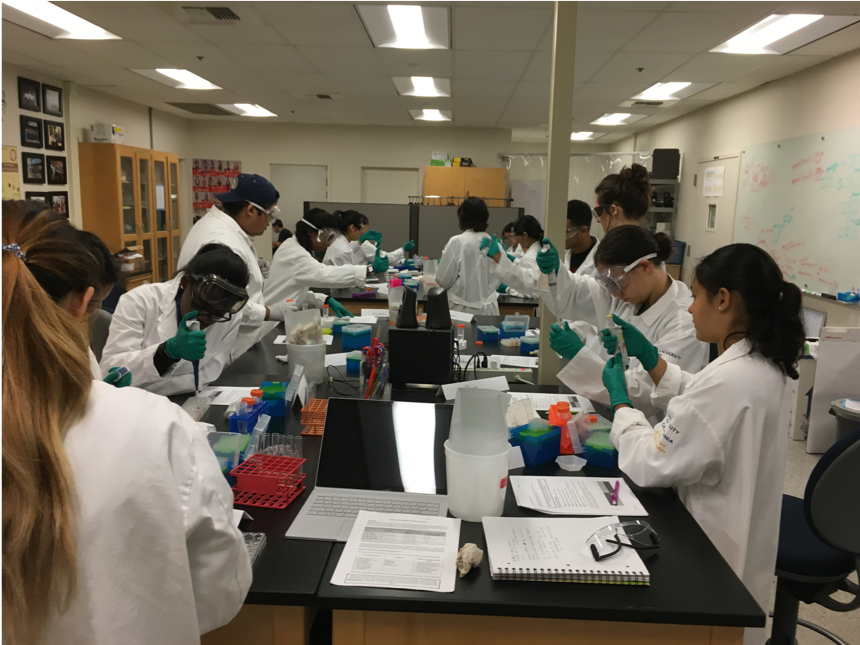
Expanding To A National Network
The results from mutant characterization increase in impact with dataset expansion and depth. We are thrilled to be further developing this project through an NSF Research Coordination Network (Award #1827246)
Incorporating undergraduate research experiences into laboratory courses vastly increases the reach and accessibility of these opportunities. The Data2Design Course-based Undergraduate Research Experience (D2D-CURE) network will train and support faculty to broaden research participation and expand high-impact educational practices. The network is designed to make this workflow accessible to a wide array of higher education institutions (community colleges, state schools, liberal arts schools, as well as research universities), as a high-impact module for existing and new biochemistry and bioengineering courses. Collectively and over time students who engage in this project will be generating datasets large enough to begin utilizing machine learning tools to improve protein design algorithms, while learning translatable skills, the process of science, and developing confidence in doing research. This network serves to both train future scientists and to advance the progress of science. The most interesting research outcomes of this project are dependent on large datasets and as such, it is optimal for multi-institutional collaborations.
We are actively seeking collaborators. Please connect with us!
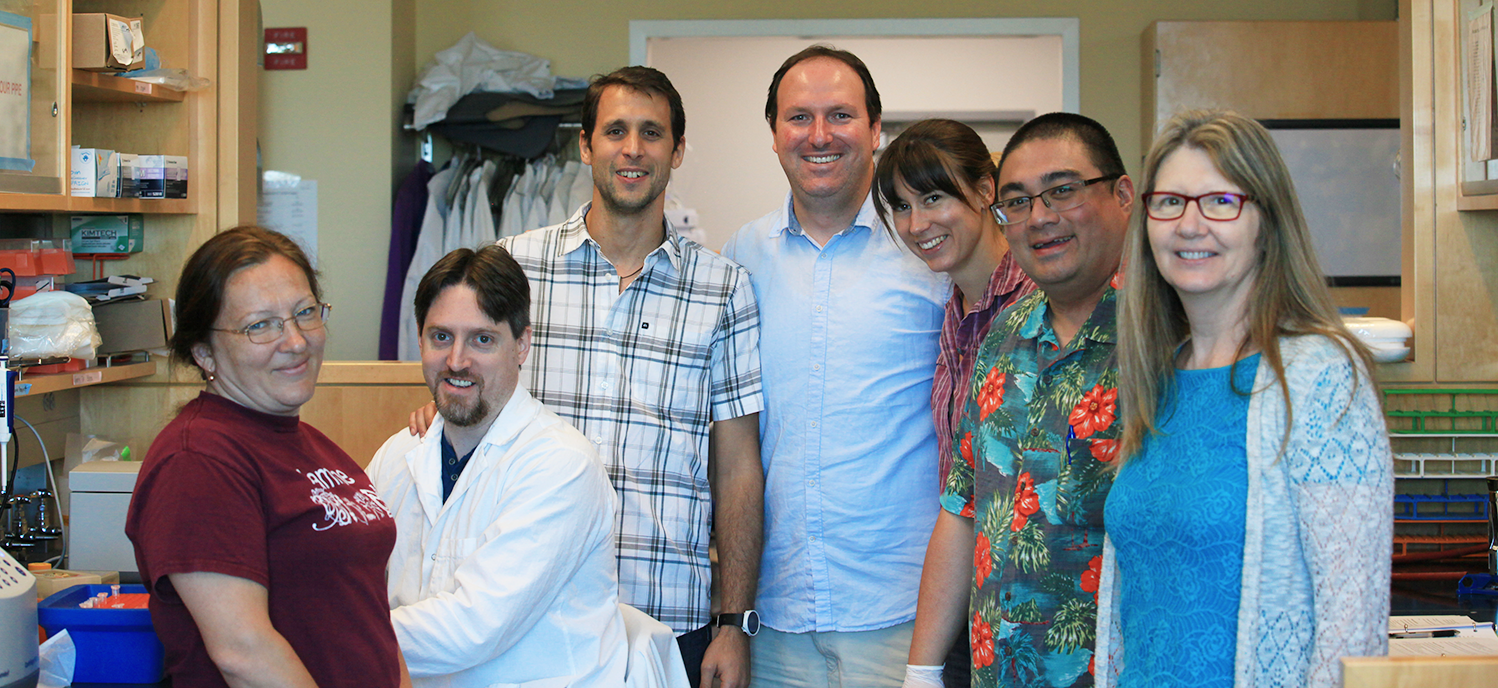